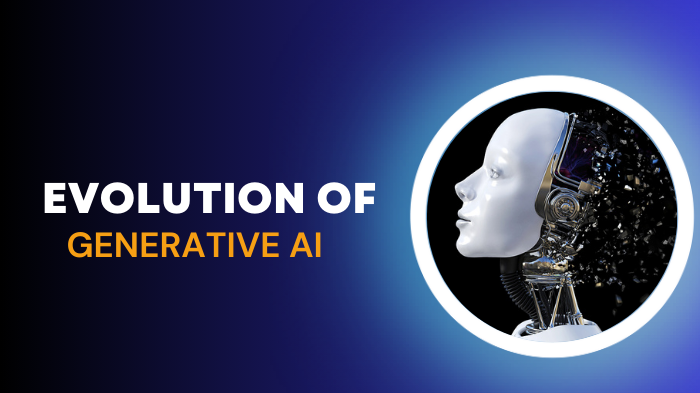
Generative AI has made remarkable strides over the past decade, transforming from a niche technological curiosity into a cornerstone of modern artificial intelligence applications. Initially focused on generating text, generative AI has now evolved into a versatile tool with multimodal capabilities, seamlessly handling tasks across text, image, audio, and video. This journey represents a profound shift in both technological sophistication and practical utility.
This blog will explore the historical milestones, technical advancements, and implications of this transformation, highlighting how generative AI has moved beyond text-based models to influence every facet of our digital lives.
The Early Days: Text-Based Generative Models
The origins of generative AI lie in natural language processing (NLP), a field dedicated to enabling machines to understand and generate human language. Early generative models were rule-based systems that relied heavily on predefined scripts and structured datasets. While effective for specific tasks, these systems were rigid and lacked creativity.
The Advent of Deep Learning
The game-changing moment for generative AI came with the adoption of deep learning techniques. Neural networks, particularly recurrent neural networks (RNNs) and later transformers, revolutionized text generation. The introduction of the sequence-to-sequence (Seq2Seq) model in 2014 enabled tasks such as machine translation and summarization, paving the way for more advanced applications.
In 2018, OpenAI released GPT (Generative Pre-trained Transformer), a language model capable of producing coherent and contextually relevant text. This marked the dawn of modern generative AI. Subsequent iterations, such as GPT-3, scaled the model to billions of parameters, unlocking unprecedented fluency and creativity.
Moving Beyond Text: Multimodal Capabilities
As the capabilities of generative AI matured, researchers sought to expand its scope beyond text. Multimodal AI refers to systems that can process and generate data across multiple types of input, such as images, audio, and video, in addition to text.
The Rise of Image Generation
The evolution of generative AI into visual domains began with the development of Generative Adversarial Networks (GANs) in 2014. GANs introduced a novel architecture comprising two neural networks—a generator and a discriminator—that competed to create realistic images. Early successes included photorealistic faces, artistic styles, and image restoration.
In 2021, OpenAI launched DALL·E, a model capable of generating high-quality images from textual descriptions. This breakthrough combined the transformer architecture with computer vision, bridging the gap between textual and visual modalities.
Audio and Speech Generation
Parallel advancements occurred in audio generation. Models like WaveNet, developed by DeepMind, demonstrated how generative AI could synthesize human-like speech and music with remarkable fidelity. Today, AI-powered tools are widely used for voice cloning, podcast editing, and background music generation.
Video and 3D Content
Video generation and 3D modeling are the newest frontiers for multimodal generative AI. These applications demand high computational resources and intricate modeling of spatial-temporal relationships. While still in its infancy, AI-generated video content is beginning to find applications in gaming, virtual reality, and filmmaking.
Core Technologies Behind Multimodal AI
Several technological breakthroughs have driven the evolution of multimodal generative AI:
- Transformer Architectures
Initially developed for text, transformer architectures now underpin most multimodal models. Vision transformers (ViTs) and extensions like CLIP (Contrastive Language–Image Pre-training) enable AI systems to understand and generate content across multiple domains. - Self-Supervised Learning
Self-supervised learning has been critical in scaling multimodal AI. By training on vast, unlabelled datasets, models can learn generalized representations applicable to diverse tasks. - Diffusion Models
Diffusion models represent a newer approach to generative tasks, excelling in high-quality image and video generation. These models iteratively refine noisy data to produce realistic outputs, a technique popularized by tools like Stable Diffusion. - Large-Scale Training
Scaling up models with billions of parameters has been instrumental in enabling multimodal capabilities. These large models can integrate information from disparate sources, achieving impressive versatility.
Applications of Multimodal Generative AI
Creative Industries
Generative AI is revolutionizing creative fields, from graphic design and video editing to music composition. Tools like DALL·E and MidJourney allow users to create stunning visual art, while AI-generated scripts and storylines enhance filmmaking and gaming.
Healthcare
In healthcare, multimodal AI assists in diagnostic imaging, drug discovery, and patient monitoring. For example, AI systems can analyze both textual patient records and medical scans to offer comprehensive insights.
Education and Accessibility
Generative AI enhances education through personalized content creation, automated tutoring, and improved accessibility for individuals with disabilities. Text-to-speech and image captioning tools are making digital resources more inclusive.
Business and Marketing
Marketers are leveraging multimodal AI to generate tailored content for advertisements, social media, and e-commerce. AI-driven personalization is improving customer engagement and boosting sales.
Challenges and Ethical Considerations
Despite its promise, the evolution of generative AI has raised critical ethical concerns.
- Bias and Fairness
Multimodal AI systems often inherit biases from their training data, which can lead to discriminatory outcomes. Ensuring fairness and transparency in AI-generated content is a pressing challenge. - Misinformation and Deepfakes
The ability to generate realistic images, audio, and videos has fueled concerns about deepfakes and misinformation. Robust detection mechanisms are essential to mitigate these risks. - Environmental Impact
Training large-scale AI models consumes significant computational resources, contributing to environmental concerns. Researchers are exploring energy-efficient techniques to reduce the carbon footprint of AI development. - Intellectual Property
The use of copyrighted material in training datasets raises questions about intellectual property rights. Balancing innovation with legal and ethical considerations remains a complex issue.
The Future of Multimodal Generative AI
As generative AI continues to evolve, several trends are likely to shape its future:
- Enhanced Multimodality
Future models will seamlessly integrate more modalities, enabling applications like real-time language translation across text, audio, and video. - Personalized AI
Generative AI will become increasingly personalized, tailoring outputs to individual preferences and contexts. - Collaborative Creativity
AI systems will serve as creative collaborators, working alongside humans to ideate, design, and execute complex projects. - Regulation and Governance
Policymakers and industry leaders will need to establish frameworks to govern the ethical use of generative AI. Transparency, accountability, and inclusivity will be key pillars.
Conclusion
The evolution of generative AI from text-based systems to multimodal powerhouses marks a transformative era in artificial intelligence. By integrating diverse data types, these models are unlocking unprecedented possibilities across industries and redefining the boundaries of creativity and automation.
While challenges remain, the continued development of multimodal generative AI promises a future where human and machine capabilities merge in novel and inspiring ways. As we stand on the cusp of this revolution, the focus must remain on harnessing the technology for the greater good, ensuring that its benefits are accessible to all.